Data mining! You might envision frightening pictures of hackers gaining access to your information or someone spying on you if you read that. However, this knowledge discovery method plays a significant and beneficial function in our daily lives and has a significant role to play in data mining for business analytics. In many countries, this mining assists professionals and researchers in learning how to assist with humanitarian operations. They can learn about disease transmission, climate change, discrimination, and other topics. Without it, getting the data we need to make forecasts and solve problems around the world would take months or years. It is used by companies all over the world for projects with a wide range of applications and meaning in the commercial sector.
This knowledge discovery role is a critical position for IT professionals, and a degree in data analytics can help you qualify for a data mining career. But, everyone in the company needs to know about it. It’s critical to know how many business processes are carried out and how information is gathered. So, existing and aspiring business professionals should be familiar with data mining and analytics insights.
This guide will teach you more about data mining, including what it is, how it works, and what it means for businesses.
Data Mining- The true meaning
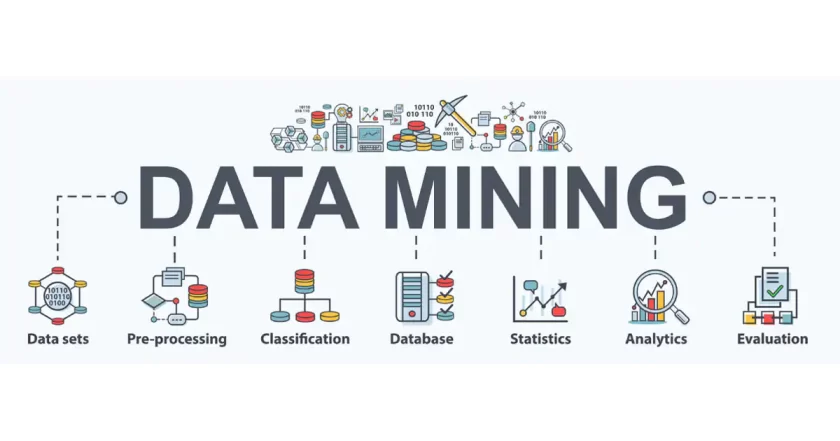
Simply put, it refers to the process that helps businesses transform unstructured data into actionable insights. They use software to hunt for patterns in massive quantities of data in order to gain a better understanding of their customers. They draw information from pooled data and compare it to support commercial decision-making. This ultimately aids them in developing plans, increasing sales, successfully marketing, and more.
The mining is frequently mistaken for machine learning and data analysis. However, the three phrases are different and distinct.
While both data mining and machine learning use patterns and analytics, data mining looks for existing patterns in data, and machine learning goes further to predict future events based on the data. The “rules” or patterns in this mining of data aren’t understood right away. In many examples of machine learning, a rule or variable is supplied to the machine to help it understand the data. Furthermore, it relies on human decision-making and interaction, whereas machine learning is designed to be started by a human and then learn on its own. There is a lot of crossover between data mining and machine learning, and machine learning algorithms are frequently used in data mining to automate procedures.
Likewise, the words data analysis and the knowledge discovery process are not interchangeable. Although both are similar, they are not the same. Data mining is the process of extracting information from massive data sets, whereas data analytics is the process of delving deeper into that information to learn more. Examining, cleansing, manipulating, and modeling data are all part of data analysis. The analysis’ ultimate purpose is to uncover usable information, draw conclusions, and make decisions.
Business intelligence procedures combine data mining (knowledge discovery), data analysis, artificial intelligence, machine learning, and a variety of other words to help a company or organization make decisions and learn more about their consumers and prospective results.
A summary of the data mining procedure
The knowledge discovery process is used by almost every business, therefore it’s critical to understand how it works and how it may assist a company makes decisions.
- Business Understanding: Understanding the overarching business objectives is the first step to successful data collection, and then being able to transform this into a data mining problem and a plan. A supermarket, let’s say, can deploy data mining techniques to understand more about its customers. A supermarket, according to conventional wisdom, is trying to figure out what its consumers are buying the most.
- Data Understanding: It’s time to collect data once you’ve figured out what the company is looking for. Data can be gathered from an organization, organized, stored, and managed in a variety of ways. Data mining entails familiarising oneself with the data, discovering any problems, gaining insights, or seeing subsets. The supermarket, for example, may implement a rewards program in which customers enter their phone numbers while making purchases, giving the supermarket access to their buying data.
- Data Preparation: Data preparation entails preparing the data for production. This is the most important aspect of data mining. It is the method of turning binary-language facts into a form that people can comprehend and measure. This step necessitates the transformation and cleaning of data in preparation for modeling.
- Modeling: Mathematical models are used to search for patterns in the data during the modeling process. For the same collection of data, there are a number of strategies that can be used. Modeling passes through a great deal of trial and error.
- Evaluation: When the model is finished, it should be thoroughly analyzed, and the procedures taken to create it should be reviewed to ensure that it fits the business objectives. A decision about the data mining results will be made at the end of this phase. The data mining results in the supermarket example will provide a list of what the customer has purchased, which is exactly what the company was searching for.
- Deployment: Depending on the process’s output, this might be a simple or sophisticated element of data mining. It could be as easy as publishing a report or as sophisticated as developing a repetitive data mining process that occurs on a regular basis.
Following the completion of the data mining process, a company will be able to make judgments and execute changes depending on what they have learned.
Data mining in business analytics
What are the benefits of mining records for businesses and their analytics? Businesses that use data mining get a competitive advantage, have a better understanding of their customers, have more control over their operations, enhance client acquisition, and discover new business prospects. This mining and analytics insight will aid diverse industries in different ways. Some industries are seeking new ways to attract new clients, while others are looking for new marketing techniques and improving current processes. The data mining process provides businesses with the tools and knowledge they need to make better decisions, analyze data, and move forward.
Now that you know why data analysis is vital, it’s time to look at how it works in business.
- Classification: This data set is more advanced, relying on data qualities to sort data into discernible groups, allowing you to draw more inferences. Supermarket data mining could use categorization to classify the various things that customers buy, such as fruit, meat, and bakery items. The store can use these classifications to understand more about its customers, outputs, and other aspects.
- Clustering: This method is similar to classification in that it groups data together based on similarities. Cluster groups are less organized than categorization groups, which makes data acquisition easier. Instead of distinct supermarket classifications, a basic cluster group of food and non-food products might be used.
- Association rules: In the collection of facts, the association is all about tracking patterns, particularly those based on connected variables. In the case of the supermarket, this could indicate that many customers who purchase one item may also purchase a second, related item. This is how stores might group similar food items together, or how online retailers can display a “people who bought this” section.
- Regression Analysis: Regression is a technique for planning and modeling that determines the likelihood of a given variable. The supermarket may be able to estimate pricing points based on availability, consumer demand, and competition. By detecting the link between variables in a set, regression aids data harvesting.
Some of the top tools for harvesting for business
- DataMelt
- Orange Data Mining
- Rattle GUI
Summary
We live in a data-driven, information-rich world. While it’s reassuring to know that there’s a wealth of information at your fingertips, the sheer volume of information can be overwhelming. The more data you have, the longer it will take to get the useful insights you require. Hence, it is important for a business to explore more and more about data mining in business analytics and its contribution to the business industry.
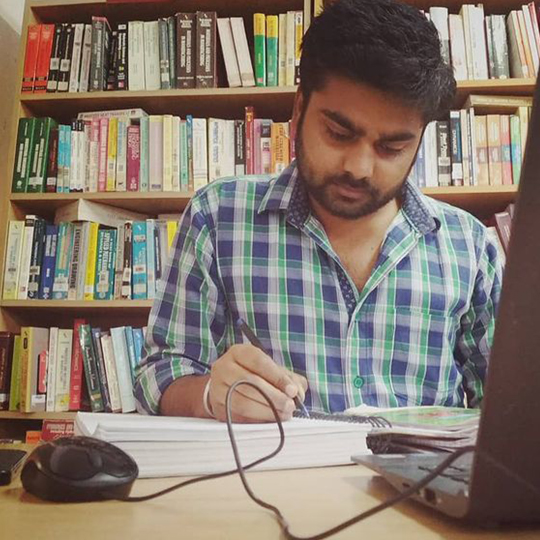
Vikas Maurya is a professional blogger and Data analyst who writes about a variety of topics related to his niche, including data analysis and digital marketing.